A Generalized Poisson-Pseudo Maximum Likelihood Estimator
CESifo, Munich, 2022
CESifo Working Paper No. 10145
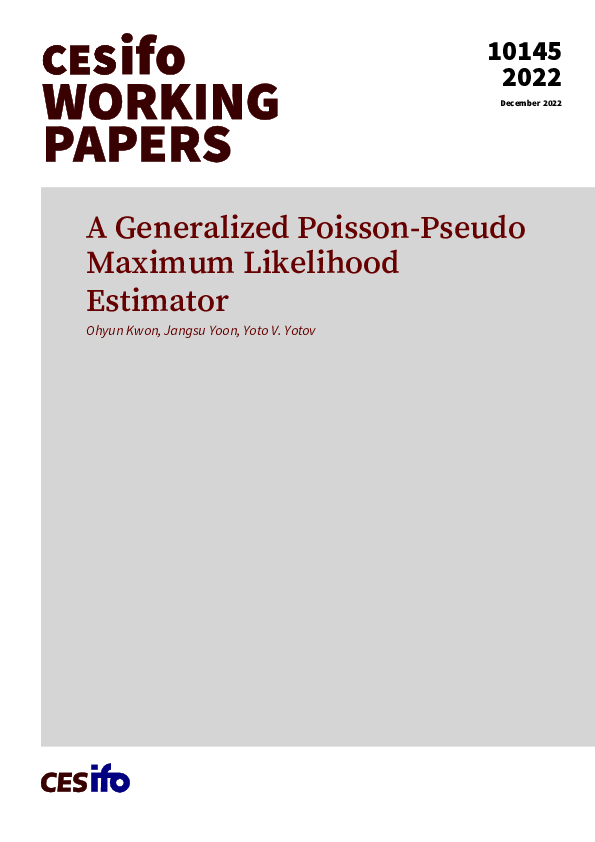
We propose a Generalized Poisson-Pseudo Maximum Likelihood (G-PPML) estimator that relaxes the PPML estimator’s assumption that the dependent variable’s conditional variance is proportional to its conditional mean. Instead, we employ an iterated Generalized Method of Moments (iGMM) to estimate the conditional variance of the dependent variable directly from the data, thus encompassing the standard estimators in international trade literature (i.e., PPML, Gamma-PML, and OLS) as special cases. With conditional variance estimates, GPPML generates coefficient estimates that are more efficient and robust to the underlying data generating process. After establishing the consistency and the asymptotic properties of the G-PPML estimator, we use Monte Carlo simulations to demonstrate that G-PPML shows decent finite-sample performance regardless of the underlying assumption about the conditional variance. Estimations of a canonical gravity model with trade data reinforce the properties of G-PPML and validate the practical importance of our methods.
Trade Policy
Empirical and Theoretical Methods